Experts lay out data illiteracy's dangers, propose remedies
In this excerpt from their new book 'Data Literacy,' top data management experts Peter Aiken and Todd Harbour outline a new way to gauge data learnedness.
In Data Literacy (2021, Technics), data management authority Peter Aiken and Todd Harbour, chief data officer for New York State, outline the levels and types of data knowledge a society and its citizens need to thrive today, plus the dangers of data illiteracy.
Editor's note: The following is condensed from the original to better fit this format.
This chapter describes the necessity of everyday citizens becoming data literate. First, we will use the COVID-19 pandemic as a collective focus for the type of problems that arise when decision makers lack an understanding of data. Then, we will define data literacy through a series of comparisons to similar skills, illustrating the need for a standardized scale for data literacy. We will describe the problem of data debt and the danger of the data matrix to the common citizen.
The pandemic illustrates the problems of a data illiterate society
We began this book in early 2020, when COVID-19 put much of our world under strict quarantine. There was much confusion, fear, opinions, defiance, hysteria … and errors. Much of the fear and confusion arose from an inability to understand what we call pandemic math. It goes like this: If the demand for beds at a 48-bed hospital doubles every day, at what point does anyone notice that the beds are growing scarce?
- Day 1: 3 beds were occupied.
- Day 2: 6 beds were occupied.
- Day 3: three-quarters of the beds were available.
- Day 4: half of the beds were available.
- Today: no beds are available.
- What should the hospital do tomorrow?
Consider the following analogy. Societies exert control over pandemics by flattening the curve associated with the rate of infection. However, if people don't understand flattening or doubling daily volumes, following the rationale for pandemic decisions will be challenging. The pandemic has shown us that data illiterate citizens cannot understand the consequences of their actions in society.
Various health officials tried to use data combinations to manage expectations throughout the pandemic [but there were problems in 2020 related to] standardizing data collection and analyses across the country.The ability to recognize change is essential if a state expects to improve its ability to measure real-world phenomena and make proper policy decisions. Everyone involved must understand data -- and information derived from the data -- to manage crises and grow in times of prosperity.
We began this book with the shocking idea that 2020 would be known as the year the AI/ML algorithms [i.e., a set of instructions or rules that, especially if given to a computer, will try to help calculate an answer to a problem] ran out of data suitable for AI training purposes! Specifically, meaningful advances in artificial intelligence (AI) depend on learning algorithms built using real-world data. In her influential book, The Big Nine, futurist Amy Webb argues that only a handful of companies do data well enough to provide training to AI programs. She makes the case that the U.S. must be a leader in the field as a matter of national security. Webb's concern underscores the real crisis, that AI algorithms are starving for data of sufficient quality to carry out the desired training. Consequently, data-needs are preventing necessary algorithm training and impeding new AI capabilities.
Using test data helps solve some of the problems, but history has shown that testing data is inadequate, incomplete, and unreliable. We saw this over the first part of the year 2020. Then, researchers presented exaggerated predictions and had to adjust their models to align with real-world measurements. Never had nations used models to set national and international policies at this scale. What many have learned is that math was not the problem. Data was the problem.
Looking back, it's clear that researchers could have built much better models using high-quality training data assets. However, those data assets didn't exist. Why? The conflict between science and individual liberty was playing out in a battle over data. This situation prevented people from effectively evaluating different approaches in a consistent and even-handed manner. This illustrates that politics and social concerns taint every measure (more data) we identify.
It is no surprise that many offered varying predictions. Until the COVID-19 coronavirus appeared, these models were just that -- models. Trouble ensued when a data illiterate media, political leadership and private citizens failed to understand the issues and data related to the various models. We contend that increasing the general population's data literacy, especially those in leadership roles, will prevent the type of data-based misunderstandings such as those that occurred in the pandemic.
The reality is that most citizens do not understand much about data. They hear people talk about data on the news, but they don't know where information comes from or how systems work with it. How do people use data? Why should I care? More importantly, citizens don't understand how data affects them on an individual level. Without that understanding, citizens can't begin to ask questions about data, let alone find the answers to their questions.
One important consequence of this is a communications bottleneck. Many are unable to consider the vast sums of storage, processing, interpretation, and more. It can be challenging to have a substantive conversation about data with someone who must make important decisions but is data illiterate.
If an individual lacks a fundamental understanding of the topic and the data, the communication can require enormous effort. Data literate participants must translate their ideas into a simple and understandable form for the decision-maker who must also engage. The data literate person must take the extra step and offer more information to help others understand data. In the end, the added effort may or may not result in effective communication. Lack of effective communication often dooms policy efforts. This task of converting or translating information falls entirely on the (always too) few data experts available. For example, suppose a data expert sees that their organization has poor "data hygiene." If the person's superiors are not data literate, trying to convince them of the need to change data processes may prove too difficult.
Data literacy is a range (as opposed to a binary measure)
Society invests significant resources in improving literacy. Education systems develop programs to be sure that our children can read and write. However, we have not yet invested similar resources in data literacy. To become more data literate, citizens need to understand the value of data and the remarkable things that we can do with it.
What is literacy?
The Data Literacy Project shows that employees with strong data literacy bring:
- 3-5% greater market capitalization
- $320-$534 million in higher enterprise value
- positive impact on margin, return-on-assets, return-on-equity and return-on-sales [according to the Data Literacy Project].
But what does data literacy entail, and how is it measured?
The Cambridge English Dictionary defines the term literate to denote a person who can read and write. When evaluating traditional literacy, we may speak of someone having a "twelfth-grade reading level" or a "second-grade reading level." This creates a range rather than a binary condition. The same must be true when describing data literacy. We believe there must be a standard scale that denotes data literacy progress. We can't accurately measure an individual's data knowledge, skills and ability across society without these measures. We define in [later in this book] a framework that permits objective specification of data knowledge optimized for differing citizen data literacy needs. These [citizen data knowledge areas] are simple and objective.
A related term is computer literacy. People apply this term to describe someone who uses electronic devices like computers, tablets and smartphones. This term's application expanded quickly to include other activities, including word processing, surfing the internet and attending online conferences. For example, consider how we apply the term computer literacy to describe someone. A computer-literate person can turn on a computer, tablet or smartphone, use it to process words, surf the internet, and place a Zoom call.
The problem with using this definition is that computer literacy initially reflected necessary operational skills but no more. For example, a computer-literate person probably has no idea that leaving equipment connected to the internet makes it susceptible to hijacking.
Foreign actors regularly commandeer citizens' computers and repurpose them for nefarious activities while citizens become unwitting agents for foreign criminals. That same "computer literate" person may be unaware that the machine is taking part in nefarious acts such as distributed denial-of-service attacks on the country's military. But as we've seen, the public often fails to realize that their behavior puts them at risk for a growing range of injuries. It is such a serious challenge that the FBI was authorized to directly remove malware from public and private MS Exchange servers in the aftermath of the SolarWinds hack.
How about financial literacy?
The concept of financial literacy has achieved a more cohesive understanding due to the coordinated efforts of professional societies and substantive funding. A typical definition of financial literacy is understanding and effectively using various financial skills, including personal financial management, budgeting and investing. The term is well understood within the profession and generally seen as a useful societal goal. Country music singer and songwriter Charlie Louvin once said it well when describing his and his brother's stage performance costumes as a tax deduction: "Of course they're deductible, but before you can deduct them from your taxes, you have to deduct 'em from your income."
If you don't appreciate the wisdom of this statement, you may benefit from increasing your own financial literacy. Clearly, Charlie Louvin was financially literate!
Like financial literacy, data literacy involves both practical and theoretical considerations.
Consider driving. Most people agree that if someone only knows how to use a car's ignition, they can't safely operate a vehicle on a public road. Turning on a car's ignition is necessary to drive a car, but it's not enough to safely drive the vehicle on public roads. Before taking a vehicle on public roadways, a person must prove an understanding of road rules. However, that's still insufficient. Student drivers also must pass a practical exam.
This test proves that a student can control the automobile under real-world conditions. Together, these tests give the government and public confidence that drivers know how to drive their vehicles safely. Successfully passing a driving test is an objective level of driving performance. This same level of performance will serve the driver well for a lifetime. This isn't to say that one cannot improve their driving abilities -- improving from driving literacy to driving acumen might improve traffic patterns.
As in the above cases, data literacy requires knowledge of basic functions (what data is) and the ability to put that knowledge to use to protect oneself and others.
Do we need "levels" of data literacy? Yes -- five of them!
Everyone produces data. Today, citizens share aspects of their lives with many others. Yet few citizens are data literate. Data illiterate citizens do not understand the consequences of their actions in society. Thus, society developed into a two-tiered system: 1) citizens interested in and becoming more data literate and 2) citizens who are not. While these represent endpoints on a range, we believe that this social dynamic will play a pivotal future role. It is difficult to imagine that the surveillance capitalists will find a profitable future with less than half of the population falling into category 2 -- it isn't practical with fewer citizens taking part. Surveillance capitalism has a personal stake in keeping data literacy rates low, a goal that is a direct attack on our fellow citizens. Increasing citizen data literacy will allow citizens to fight to keep and monetize their own data rather than allowing others to profit from it.
It is easy to declare someone as data literate, but it is another thing to prove it. This stems from trying to precisely define the single term, data literacy. Ordinary interpretation suggests that data literacy is someone capable of [reading, analyzing, and using data]. Unfortunately, it is not useful as a measure as it lacks objective standards. As a result, citizens make self-assessments about their data literacy, and society has no way to confirm or refute those claims. This contrasts with other disciplines such as reading, in which we have ways to measure a person's competency through objective measures.
We believe proving someone's data literacy isn't a binary assertion -- either literate or illiterate. Instead, we should measure data literacy against a range reflecting objectively measurable aspects. The range moves from data literacy to data proficiency to data acumen, just as children move from spelling "cat" and "dog" at a kindergarten level to writing multi-page research essays at the twelfth-grade level.
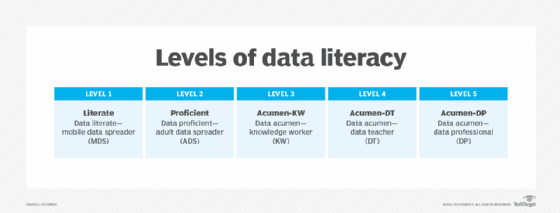
Each ranking level represents an objectively higher level of data competency. The measure allows us to make objective assertions about someone's data competencies at each point along the scale. This kind of tool has many different uses. For example, it would allow employers to discriminate among applicants, tailor position descriptions and compare applicants.
[Later in this book we] describe these levels more precisely in the context of a Digital Civics Framework. For now, it is sufficient to understand that these five levels more accurately and objectively reflect the challenge associated with improving citizen data literacy and achieving a more manageable understanding of facts (data values).