supply chain analytics
What is supply chain analytics?
Supply chain analytics refers to the processes organizations use to gain insight and extract value from the large amounts of data associated with the procurement, processing and distribution of goods. Supply chain analytics is an essential element of supply chain management (SCM).
The discipline of supply chain analytics has existed for more than 100 years, but the mathematical models, data infrastructure and applications underpinning these analytics have evolved significantly. Mathematical models have improved with better statistical techniques, predictive modeling and machine learning. Data infrastructure has changed with cloud infrastructure, complex event processing (CEP) and the internet of things (IoT). Applications have grown to provide insight across traditional application silos such as enterprise resource planning (ERP), warehouse management, logistics and enterprise asset management.
An important goal of supply chain analytics software is to improve forecasting and efficiency and be more responsive to customer needs. For example, predictive analytics on point-of-sale terminal data stored in a demand signal repository can help a business anticipate consumer demand, which in turn can lead to cost-saving adjustments to inventory and faster delivery.
Achieving end-to-end visibility in supply chain analytics entails researching the procurement of raw materials and extends through production, distribution and aftermarket services. This depends on effective integration with the many SCM and supply chain execution platforms that make up a typical company's supply chain. The goal of such integration is supply chain visibility -- the ability to view data on goods at every step in the supply chain.
Most industries require supply chains to create what they produce and sell. The manufacture of cell phones, for instance, requires the purchase of many components -- each of which often has its own supply chains -- that make their way to a factory by supply chain, often from other companies, across many modes of transportation. This transport of materials must be carefully optimized to accommodate complex assembly schedules to promptly accommodate consumer demand, at which point the cell phones themselves enter the supply chains of retailers and other distributors. Disruptions in the supply chain can upset the production schedule and can result in a failure to meet product demand.
Supply chain analytics software
Supply chain analytics software is generally available in two forms: embedded in supply chain software, or in a separate, dedicated business intelligence and analytics tool that has access to supply chain data. Most ERP vendors offer supply chain analytics features, as do vendors of specialized SCM software. Some IT consultancies also develop software models that can be customized and integrated into a company's business processes.
Some ERP and SCM vendors have begun applying CEP to their platforms for real-time supply chain analytics. Most ERP and SCM vendors have one-to-one integrations, but there's no standard. However, the supply chain operations reference, or SCOR model, provides standard metrics for comparing supply chain performance to industry benchmarks.
Ideally, supply chain analytics software would be applied to the entire chain, but in practice, it's often focused on key operational subcomponents, such as demand planning, manufacturing production, inventory management or transportation management. For example, supply chain finance analytics can help identify increased capital costs or opportunities to boost working capital; procure-to-pay analytics can help identify the best suppliers and provide early warning of budget overruns in certain expense categories; and transportation analytics software can predict the impact of weather on shipments.
How supply chain analytics works
Supply chain analytics brings together data from different applications, infrastructure, third-party sources and newest technologies, such as IoT, to improve decision-making across the strategic, tactical and operational processes that make up supply chain management. Supply chain analytics helps synchronize supply chain planning and execution by improving real-time visibility into these processes and their impact on customers and the bottom line. Increased visibility can also increase flexibility in the supply chain network by helping decision-makers to better evaluate tradeoffs between cost and customer service.
The process of creating supply chain analytics typically starts with data scientists who understand a particular aspect of the business, such as the factors that relate to cash flow, inventory, waste and service levels. These experts look for potential correlations between different data elements to build a predictive model that optimizes the output of the supply chain. They test out variations until they have a strong model.
Supply chain analytics models that reach a certain threshold of success are deployed into production by data engineers with an eye toward scalability and performance. Data scientists, data engineers and business users work together to refine the way these data analytics are presented and operationalized in practice. Supply chain models are improved over time by correlating the performance of data analysis models in production with the business value they deliver.
Features of supply chain analytics
Supply chain analytics software generally includes the following features:
- Data visualization. Supply chain analysts can slice and dice data from different angles to improve insight and understanding.
- Stream processing. This feature derives insight from multiple data streams generated by, for example, the IoT, applications, weather reports and third-party data.
- Social media integration. Analysts can improve demand planning by using sentiment data from social feeds.
- Natural language processing. Analysts can extract and organize unstructured data buried in documents, news sources and data feeds.
- Location intelligence. Analysts derive insight from location data to understand and optimize distribution.
- Digital twin of the supply chain. Analysts organize data into a comprehensive model of the supply chain that's shared across different types of users to improve predictive and prescriptive analytics.
- Graph databases. Analysts organize information into linked elements that make it easier to find connections, identify patterns and improve traceability of products, suppliers and facilities.
Types of supply chain analytics
A common lens used to delineate the main types of supply chain analytics is based on Gartner's model of the four capabilities of analytics: descriptive, diagnostic, predictive and prescriptive.
- Descriptive supply chain analytics uses dashboards and reports to help interpret what has happened. It often involves using a variety of statistical methods to search through, summarize and organize information about operations in the supply chain. This can be useful in answering questions like, "How have inventory levels changed over the last month?" or "What is the return on invested capital?"
- Diagnostic supply chain analytics are used to determine why something happened or isn't working as well as it should. For example, "Why are shipments being delayed or lost?" or "Why is our company not achieving the same number of inventory turns as a competitor?"
- Predictive supply chain analytics helps to foresee what is likely to happen in the future based on current data. For example, "How will new trade regulations or a pandemic lockdown affect the availability and cost of raw materials or goods?"
- Prescriptive supply chain analytics helps prescribe or automate the best course of action using optimization or embedded decision logic. This can help improve decisions about when to launch a product, whether to build a factory or the best shipment strategy for each retail location.
Another way of breaking down types of supply chain analytics is by their form and function. Advisory firm Supply Chain Insights, for example, breaks down the types of supply chain analytics into the following functions:
- Workflow.
- Decision support.
- Collaboration.
- Unstructured text mining.
- Structured data management.
In this model, the different types of analytics feed into each other as part of an end-to-end ongoing process for improving supply chain management.
For example, a company could use unstructured text mining to turn raw data from contracts, social media feeds and news reports into structured data that's relevant to the supply chain. This improved, more structured data could then help automate and improve workflows, such as procure-to-pay processes. The data in digitized workflows is much easier to capture than data from manual workflows, thus increasing the data available for decision support systems. Better decision support could in turn enhance collaboration across different departments like procurement and warehouse management or between supply chain partners.
Other technologies are emerging as ways to improve the predictive models generated by supply chain analytics. For example, organizations are starting to use process mining to analyze how they execute business processes. This type of process analytics can be used to create a digital twin of the organization that can help identify supply chain opportunities for automation across procurement, production, logistics and finance. Augmented analytics can help business users ask questions about the business in plain language, with responses delivered in summaries. Graph analytics can shed light on the relationships between entities in the supply chain, such as how changes in a tier 3 supplier might affect tier 1 suppliers.
Supply chain analytics uses
Sales and operations planning uses supply chain analytics to match a manufacturer's supply with demand by generating plans that align daily operations with corporate strategy. Supply chain analytics is also used to do the following:
- Boost margins and cut costs by leveraging visibility of real-time data to spot inefficiencies, discrepancies and savings opportunities.
- Improve risk management by identifying known risks and predicting future risks based on patterns and trends throughout the supply chain.
- Increase planning accuracy by analyzing customer data to identify factors that increase or decrease demand.
- Improve order management by consolidating data sources to assess inventory levels, predict demand and identify fulfillment issues.
- Streamline procurement by organizing and analyzing spending across departments to improve contract negotiations and identify opportunities for discounts or alternative sources.
- Increase working capital by improving models for determining the inventory levels required to ensure service goals with minimal capital investment.
Tools for supply chain analytics
There are numerous tools supply chain analysts can use for ad hoc analysis, decision-making and problem-solving. Examples of these tools are listed below in unranked, alphabetical order:
- Aptos Enterprise Analytics.
- IBM Cognos Analytics.
- Infor Birst.
- Oracle PeopleSoft Supply Chain Analytics.
- Qlik Supply Chain Analytics.
- SAP Supply Chain Analytics.
- SAS Supply Chain Intelligence.
- Tableau Software Supply Chain.
- Tibco Spotfire.
History of supply chain analytics
Supply chain analytics has its roots in the work of Frederick Taylor, whose 1911 publication, The Principles of Scientific Management, laid the groundwork for the modern fields of industrial engineering and supply chain management. Henry Ford adopted Taylor's techniques in the creation of the modern assembly line and a supply chain that supported more efficient means of production.
The advent of mainframe computers gave rise to the data processing work done by IBM researcher Hans Peter Luhn, who some credit for coining the term business intelligence in his 1958 paper, "A Business Intelligence System." His work helped build the foundation for the different types of data analytics used in supply chain analytics.
In 1963, Dr. Bernard J. "Bud" La Londe, a professor at Ohio State University, proposed that physical distribution management should be combined with materials management, procurement and manufacturing into what he called business logistics. Around this time, management consultant Stafford Beer and others began exploring new ideas like the viable systems model for organizing business information into a structured hierarchy to improve business planning and execution. By the early 1980s, the burgeoning field was known as supply chain management.
As the internet became a force in the 1990s, people looked at how it could be applied in supply chain management. A pioneer in this area was British technologist Kevin Ashton. As a young product manager tasked with solving the problem of keeping a popular lipstick on store shelves, Ashton used radio frequency identification (RFID) sensors to automatically capture data about the movement of products across the supply chain. Ashton, who went on to cofound the Massachusetts Institute of Technology's Auto-ID Center that perfected RFID technology and sensors, coined the term internet of things to explain this revolutionary new feature of supply chain management.
The 1990s also saw the development of CEP by researchers such as the team headed by Stanford University's David Luckham and others. CEP's ability to capture incoming data from real-time events helped supply chain managers correlate low-level data related to factory operations, the physical movements of products and weather into events that could then be analyzed by supply chain analytics tools. For example, data about production processes could be abstracted into factory performance, which in turn could be abstracted into business events related to things like inventory levels.
Another turning point in the field of supply chain analytics was the advent of cloud computing, which provides a vehicle for delivering IT infrastructure, software and platforms as a service. By providing a foundation for orchestrating data across multiple sources, the cloud has driven improvements in many types of analytics, including supply chain analytics. The emergence of data lakes like Hadoop let enterprises capture data from different sources on a common platform, further refining supply chain analytics by letting companies correlate more types of data. Data lakes also made it easier to implement advanced analytics that operated on a variety of structured and unstructured data from different applications, event streams and the IoT.
In recent years, robotic process automation -- software that automates rote computer tasks previously performed by humans -- has become a powerful tool in improving business automation and the ability to integrate data into analytics.
In addition, the artificial intelligence (AI) technique known as deep learning is increasingly being used to improve supply chain analytics. Deep learning techniques are being used to drive advances in machine vision such as inventory tracking and natural language understanding is being used to automate contract management and improve routing models.
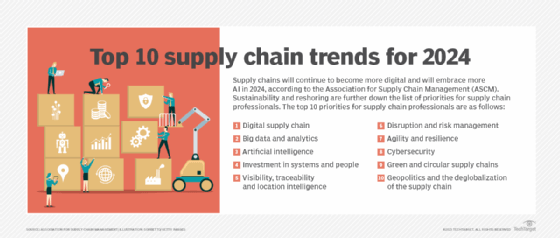
Future trends of supply chain analytics
Supply chain analytics will continue to evolve in tandem with analytics models, data structures and infrastructure, enabling organizations to integrate data across application silos. Eventually, advanced analytics will lead to more autonomous supply chains that can manage and respond to changes, much like self-driving cars are starting to do today. In addition, improvements in IoT, CEP and streaming architectures will let enterprises derive insight more quickly from a larger variety of data sources. AI techniques will continue to improve users' ability to generate more accurate and useful predictive insights that can be embedded into workflows.
Other technologies expected to play a big role in supply chain analytics and management include the following:
- Blockchain. Blockchain infrastructure and technologies promise to improve visibility and traceability across more layers of the supply chain. These same building blocks could drive companies to use smart contracts to automate, control and execute transactions.
- Graph analytics. Graph analytics will help supply chain managers better analyze the links of various entities in the supply chain by combining data sources such as event data and supply chain data.
- Hyperautomation. The technologies underpinning hyperautomation will accelerate supply chain automation by using process mining analytics to identify automation candidates, generate the automations and manage these automated processes.
Learn how generative AI can help organizations improve their key supply chain processes.